In this paper, we address the problem of searching action proposals in unconstrained video clips. Our approach starts from actionness estimation on frame-level bounding boxes, and then aggregates the bounding boxes belonging to the same actor across frames via linking, associating, tracking to generate spatial-temporal continuous action paths. To achieve the target, a novel actionness estimation method is firstly proposed by utilizing both human appearance and motion cues. Then, the association of the action paths is formulated as a maximum set coverage problem with the results of actionness estimation as a priori as in [1]. To further promote the performance, we design an improved optimization objective for the problem and provide a greedy search algorithm to solve it. Finally, a tracking-by-detection scheme is designed to further refine the searched action paths. Extensive experiments on two challenging datasets, UCF-Sports and UCF-101, show that the proposed approach advances state-of-the-art proposal generation performance in terms of both accuracy and proposal quantity.
|
|
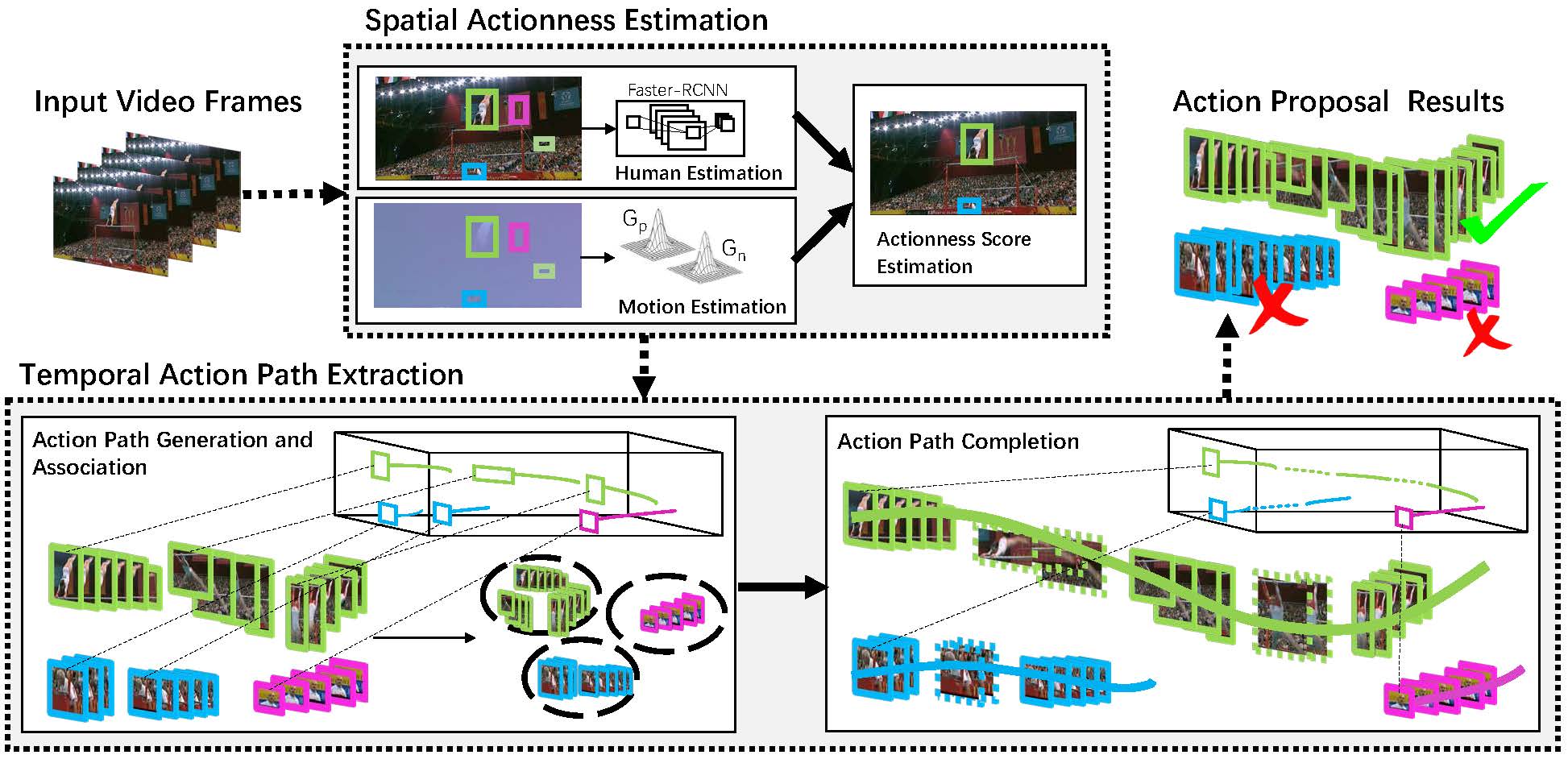